Driver gaze motions are one of the primary clues to understanding intentions behind the wheel and evaluating the acceptability and usability of HMI.Professor Takahiro Tanaka, Nagoya University Institutes of Innovation for Future Society, HMI and Human Characteristics Research Div.
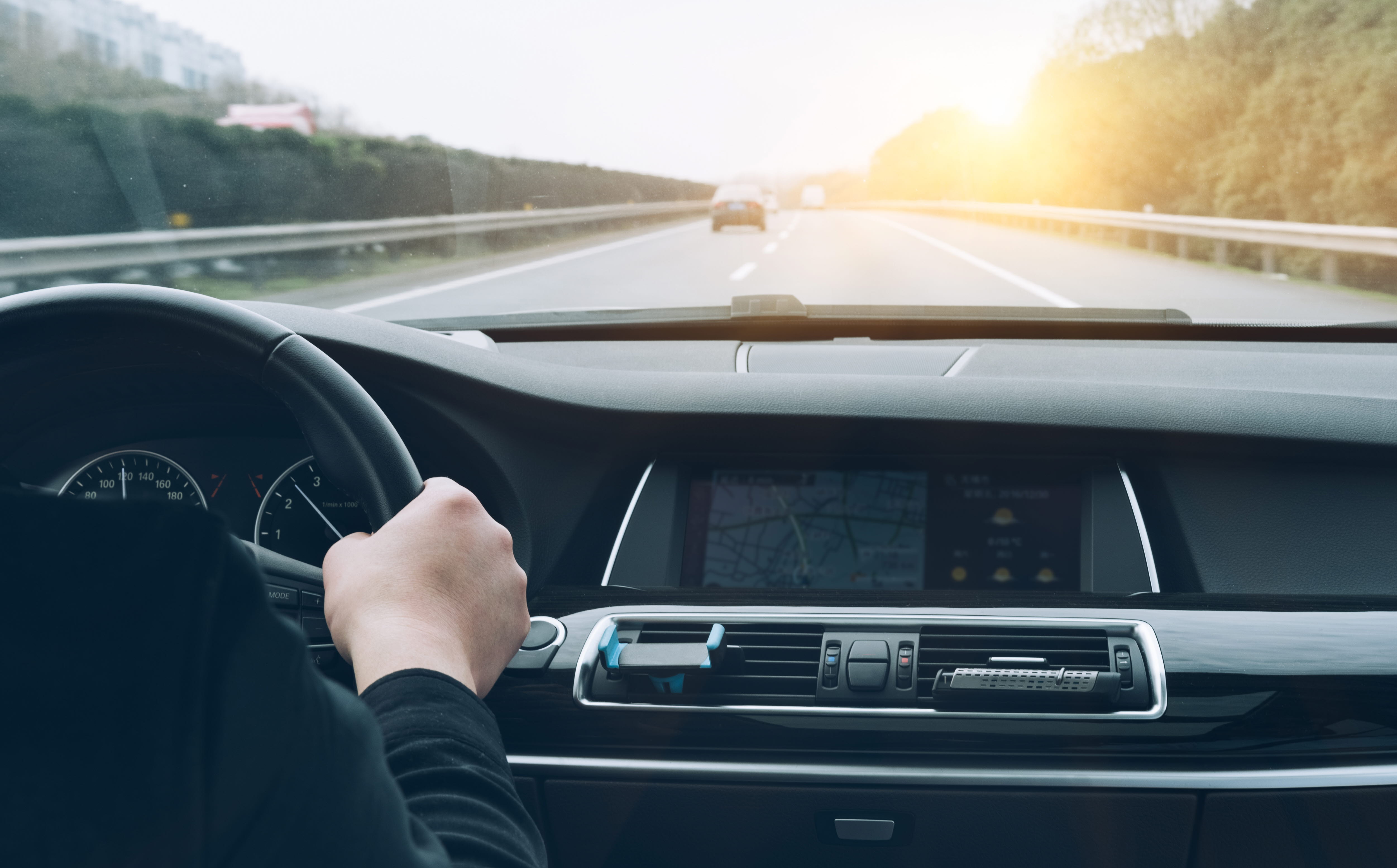
事例
Understanding human behavioral mechanisms to benefit new technology
名古屋大学 未来社会創造機構
カテゴリー詳細
執筆者
Tobii
読了時間
6 min
At Nagoya University’s Institutes of Innovation for Future Society, forward thinking researchers are exploring how human behavioral mechanisms can be understood to benefit new technology.
The question
At the Institutes of Innovation for Future Society at Nagoya University, the HMI and Human Characteristics Research Division studies interaction design between humans and intelligent systems for novel mobility interfaces and services. These researchers are working to introduce new interfaces and services based on the analysis of driver behaviors behind the wheel. Eye tracking solutions empower their researchers to recognize crucial gaze motions of drivers without any intrusion.
The method
In Japan, road accidents caused by elderly drivers are increasing. On top of this, cars are becoming ever more crucial for their continued independence in their daily lives. This is why the Institute for Innovation for Future Society, Nagoya University, used a study to propose a driver-agent system that supports elderly car users and encourages them to improve their driving in general.
In the eye tracking study, the Nagoya University laboratory gathered thirty male and female drivers between the ages of 50 and 76 to observe how they perform within a driving simulator when approaching a stop sign at an intersection. The data drawn from this analysis was able to show visual information processing abilities, awareness allocation function, and field of view.
Researchers experimented with the use of three distinct driver agents: a vocal, a visual, and a robot agent. These were applied in simulators, set-up with Tobii eye trackers, offering the same support to elderly drivers in order to determine the most effective version. The simulator presented a driving situation with a high collision rate amongst elderly drivers, and the different agents provided driving guidance through the danger zone. The eye trackers recorded gaze data for insight into attention driver distraction.
The conclusion
Overall, results of the study showed that, contrary to initial hypotheses the robot driver agent was the most effective at encouraging safe driving behaviors and preventing accidents. It was the most noticeable, least intrusive, and most acceptable to the majority of elderly drivers. Fixation points were most converged in the robot condition. and gaze time corresponded to a very short duration, implying that a similar agent does not necessarily lead to huge driving disturbance.
The vocal-only agent was the least helpful for elderly drivers despite the assumption that it would be the easiest to notice. It caused the most diverged gaze patterns, possibly indicating that drivers couldn’t understand information being provided and instinctively searched to find the source.
参考文献
Takahiro Tanaka, Kazuhiro Fujikake, Takashi Yonekawa, Misako Yamagishi, Makoto Inagami, Fumiya Kinoshita, Hirofumi Aoki, Hitoshi Kanamori (2018).
Study on Driver Agent based on Analysis of Driving Instruction Data - Driver Agent for Encouraging Safe Driving Behavior (1)
Takahiro Tanaka, Kazuhiro Fujikake, Takashi Yonekawa, Makoto Inagami, Fumiya Kinoshita, Hirofumi Aoki, Hitoshi Kanamori (2018).
Takahiro Tanaka, Kazuhiro Fujikake, Yuki Yoshihara, Nihan Karatas, Hirofumi Aoki, Hitoshi Kanamori (2020)
Takahiro Tanaka, Kazuhiro Fujikake, Yuki Yoshihara, Nihan Karatas, Kan Shimazaki, Hirohumi Aoki, Hitoshi Kanamori (2020).
カテゴリー詳細
執筆者
Tobii
読了時間
6 min
カテゴリータイプ
対象製品
対象別ソリューション
共有する
関連コンテンツ
ドライバーのためのデザイン設計
自動車メーカーがアイトラッキングを活用して、運転中の快適さと安全性をどのように確保しているか。
Using eye movements to understand driving behavior and user experience
This research spotlight focuses on two esteemed researchers as they present their work in areas where eye movement data has been applied - driving and user experience.